01-智能客服 V0.1
你好,我是悦创。
相关信息
迷时师渡,悟了自渡。「是度还是渡」。
- 纯实战,纯代码,讲落地。
- 一个项目,递进式地为你深入浅出。
1. 本节大纲
- 对话机器人的产品设计, 10分钟
- 大语言模型的使用, 30分钟
- 提示词工程,20分钟
- 开发环境讲解,10分钟
- 工程化代码讲解,35分钟
- 答疑和总结,15分钟
2. 安装库
安装 openai、pandas、tiktoken。
# 这里是依赖库,运行代码前需要先安装
!pip install openai pandas tiktoken
3. 早期的对话系统
早期,没有大语言模型的时候,我们对话系统是如何实现的?
def AssistantResponse(user_message):
if user_message in ["你好", "Hello", "Bonjour"]:
return "欢迎您!"
elif user_message in ["你是谁", "你叫什么名字"]:
return "我是机器人小悦"
else:
return "不好意思,我没能理解您的问题"
简单的调用进行体验一下:
user_message = "你好"
print(f'User: {user_message}\nAssistant: {AssistantResponse(user_message)}')
输出:
User: 你好
Assistant: 欢迎您!
我们可以再进行测试:
user_message = "你是"
print(f'User: {user_message}\nAssistant: {AssistantResponse(user_message)}')
输出:
User: 你是
Assistant: 不好意思,我没能理解您的问题
继续测试:
user_message = "你是谁"
print(f'User: {user_message}\nAssistant: {AssistantResponse(user_message)}')
输出:
User: 你是谁
Assistant: 我是机器人小悦
所以,你应该到这里能发现。我们现在的机器人🤖能不能正常回答,取决于:我们有没有提前预判用户可能会问的问题🙋。这种情况下,按目前的代码,只能使用穷举法。——但是,实际上是不可能的,一种语言都做不完。何况,有各类语言。用户换一种提问方式,机器人也会失效,所以早期这种实现是有很大局限性的。
中间还经历过各种不同技术驱动的系统,例如最经典的 RASA ...意图识别,技能,填槽,动作
4. 大模型初体验
import openai
# openai.api_key = 'Raplace to your API Key'
openai.api_key = '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'
prompt = ["问题:介绍一下 AI悦创·编程一对一是什么类型的公司\n回答:"]
response = openai.Completion.create(engine="text-davinci-002", prompt=prompt, temperature=0, max_tokens=1024)
print(response['choices'][0]['text'])
输出:
AI悦创·编程一对一是一家专注于为学生提供个性化编程教育的公司。我们提供针对性的课程设置和专业的师资队伍,帮助学生学习编程,培养创新能力和解决问题的能力。
可以看见,上面大语言模型的回答并不是那么合适。会给你一个虚假的回答,也就是不懂装懂。所以,我们也需要自己拥有辨别能力。
我们,可以通过提示工程或者向量数据库等,都可以进行解决。
5. 小悦技术全景图
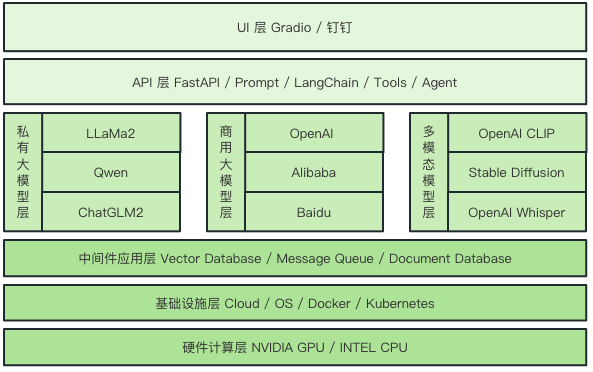
首先来看就是我们小悦技术架构图怎么来设计。
首先的话就是我们无论做怎样的软件系统,它都是需要有一个硬件把这个东西跑起来。我们实际上会用到 OpenAI 的 GPT 的模型,也会用到我们私有化部署的 LLaMa2 的模型,还有各种各样的 AI 模型。
那这些模型,有些是需要通过本地私有化的方式来部署,那就需要用到 GPU 了。那上层的话可能就是我们要做服务交付的时候,会用到容器相关的技术。那就会用到云计算相关的算力资源,然后还有像 docker 这种容器管理的工具和平台。
在最上层,我们利用各种中间件来解决特定的问题,例如前面提到的三种数据库。中间层则涵盖了各种大型模型,如 LAMA Two、Checker M2、OpenAI 的 GPT 模型,以及我们先前使用的 Tax Da Vinci 02 等。而在最底层,我们将进行大量的工程封装,将这些模型转化为 API,并通过UI进行简洁的展示。简而言之,这就是我们课程的核心内容,并且可以被概括为一张图。
6. 小悦关键流程图
提示
叫小悦还是小墨我还在,纠结中。期待你的建议。
其实,提到如 OpenAI GPT或者拉玛这样的技术,我相信大家都不陌生。但具体的实现流程是怎样的呢?其实非常直接:只要用户提出问题,系统便会给出答复,就这么简单。
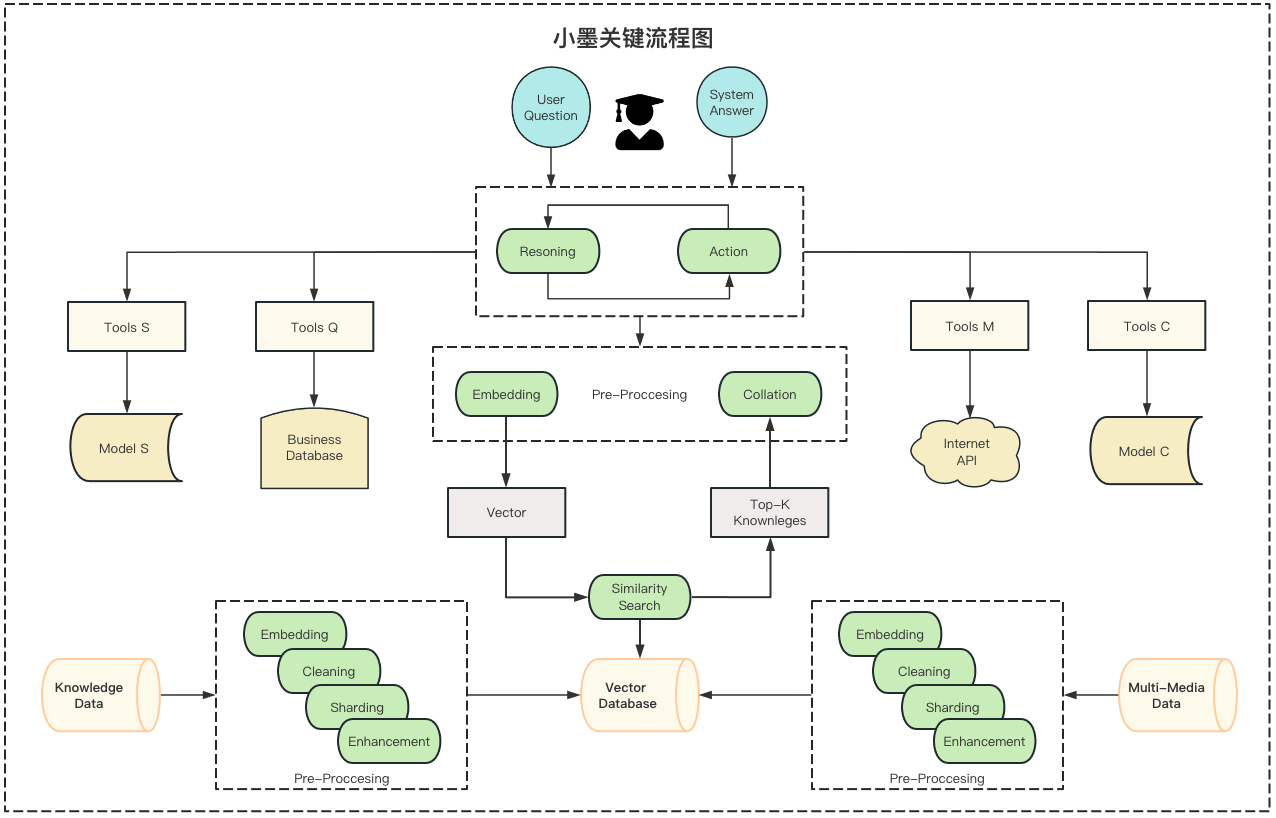
当然,这是一个基本的流程。
但在实际应用中,我们会增加很多复杂性。为什么要这样做?因为简单的流程容易导致我们最初展示的那种幻觉「答非所问」问题。针对这个幻觉问题,之前我们提到过有多种解决方法。而在这门课程中,我们会采用这些方法来逐一解决这个问题。
首先,我们可以采用向量数据库的方法或者微调模型来减少幻觉的发生。这涉及到许多核心技术,例如,如何最大化地利用 OpenAI 的模型以获得最佳效果。此外,如何微调一个模型或使用向量数据库来解决特定问题都是充满挑战的话题。
这门课程涵盖的内容,只要大家能够掌握,无疑可以助你们实现个人目标,包括升职加薪等。
我们已经详细介绍了不少内容。那么,如何实现“小悦 V0.1”呢?
我们的目标是找到一个最简单的方法,使“小悦V0.1”这个版本得以实现。实际上,我们刚刚深入讨论了一个最基础的系统,用户只需提交一个问题,系统便会为其提供答复。整个过程是由一个大型模型驱动的。在实现过程中,我们还会在 UI 上使用 gradio,同时借助 LangChain 来搭建服务,利用某个开发框架进行中间过程的开发,再用 Jupyter 来调试我们的代码。
7. 小悦 V0.1 介绍
幻觉的存在导致回复的不是我们想要的,小悦对话机器人应运而生,开始打造 V0.1 版本
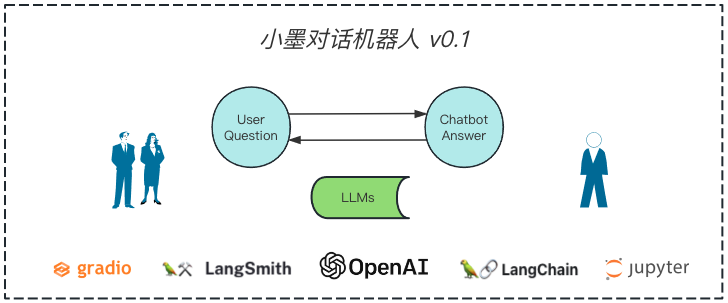
- 得封装下服务来调用下大语言模型;
- 需要了解下大语言模型如何调用;
- 需要有一个前端来给用户操作;
- 需要知道用户使用的好不好;
- ...
“POC”是“Proof Of Concept”的缩写,中文常译为“概念验证”。这是一个实验或原型,其目的是验证某个想法、概念或理论在实际应用中是否可行。通过POC,开发者或研究者可以验证某个解决方案在特定场景中是否有效,从而避免在完整开发之前浪费时间和资源。
在我上面写出:“总而言之,先做一个 POC 来验证下是可行性”就是说,在全面开发或实施之前,先制作一个原型或实验来确认这个想法或方法是否真的可行。
8. LLM 是一个关键,了解下 OpenAI
9. 开始项目
9.1 UI
我们要实现简单的对话,就需要一个 UI。那 UI 如何解决呢?——我们直接使用 Gradio。
直接 copy 代码即可。
# pip install https://gradio-builds.s3.amazonaws.com/1265a9ac134f867f4187c1f7d15ab27dbdf7503b/gradio-3.47.1-py3-none-any.whl
pip install https://gradio-builds.s3.amazonaws.com/1d986217f6f4fc1829e528d2afe365635788204f/gradio-4.1.1-py3-none-any.whl
import gradio as gr
import random
import time
with gr.Blocks() as demo:
chatbot = gr.Chatbot()
msg = gr.Textbox()
clear = gr.ClearButton([msg, chatbot])
def respond(message, chat_history):
bot_message = random.choice(["How are you?", "I love you", "I'm very hungry"])
chat_history.append((message, bot_message))
time.sleep(2)
return "", chat_history
msg.submit(respond, [msg, chatbot], [msg, chatbot])
if __name__ == "__main__":
demo.launch()
接下来,直接运行即可,效果如下:
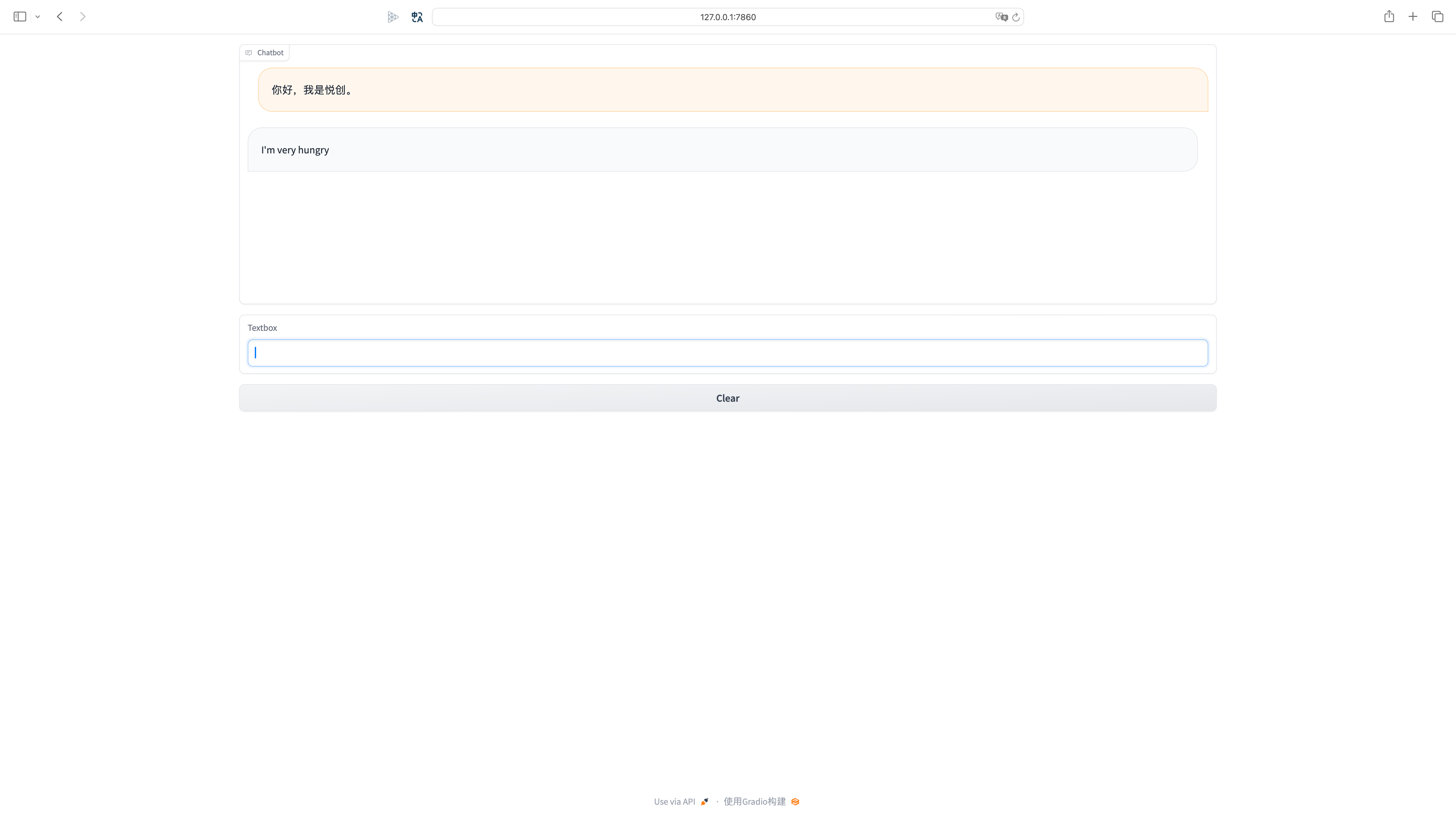
注意
我们不需要过多关心这个 UI 是如何实现的,我们只需要能用就行。
不过,我们可以稍微了解代码的部分实现要点:
bot_message = random.choice(["How are you?", "I love you", "I'm very hungry"])
主要实现随机回复,并合并成元组一并提交。
chat_history.append((message, bot_message))
这部分也是我们后面实现的重点。
time.sleep(2) ,# 假装为 Ai 真的在回复,很有趣的小点
相关信息
想想🤔用户输入的信息,存储在哪个变量?——你会怎么测试或者观察出来呢?
9.2 web.py
我们来看看我们的代码入口 web.py
:
import gradio as gr
import service
s = service.Service()
with gr.Blocks() as demo:
gr.HTML("""<h1 align="center">小悦 v0.1 - 纯 LLM 驱动</h1>""")
chatbot = gr.Chatbot()
msg = gr.Textbox()
clear = gr.ClearButton([msg, chatbot])
def respond(message, chat_history):
bot_message = s.simple_answer(message, chat_history)
chat_history.append((message, bot_message))
return "", chat_history
msg.submit(respond, [msg, chatbot], [msg, chatbot])
if __name__ == "__main__":
demo.launch(share=True, server_name="0.0.0.0")
这里,较于之前代码来说,其实是有区别的。主要区别在于 16 行,我是改成自己设计的回复。
接着,我们去看看我们 service.py
是如何实现的?
9.3 service.py
import prompt
import util
import config
class Service:
def __init__(self):
super(Service, self).__init__()
self.util = util.Util()
self.configs = config.ConfigParser()
def simple_answer(self, message, history):
# 1.组装系统提示,历史对话,用户当前问题
system_prompt = prompt.SIMPLE_SYSTEM_PROMPT
messages = self.util.concat_chat_message(system_prompt, history, message)
# 2. 去调用 OpenAI 的接口完成任务
response = self.util.ChatOpenAI(messages)
return response.content
9.4 LLM 是一个关键,了解下 OpenAI
OpenAI 提供了很多模型,都可以通过 API 调用。
如果遇到:OpenAI‘s services are not available in your country
window.localStorage.removeItem(Object.keys(window.localStorage).find(i=>i.startsWith('@@auth0spajs')))
复制这段 JavaScript 代码,然后浏览器 F12 进入调试模式,在 Console 对话框粘贴并执行
9.4.1 查看模型名称
查看 OpenAI 提供了什么模型可以使用,这样我们才知道什么具体的模型可以使用。
注意
请注意这节课,我们只会用到 Language Model
import pandas as pd
# openai.Engine.list()['data'] # 返回 json
pd.DataFrame(openai.Engine.list()['data'])
# 查看 OpenAI 提供了什么模型可以使用
# 请注意这节课,我们只会用到 Language Model
import pandas as pd
# 设置Pandas以便能显示更多信息
pd.set_option('display.max_columns', None) # 无限制显示列
pd.set_option('display.max_rows', None) # 无限制显示行
pd.set_option('display.max_colwidth', None) # 显示完整的列内容
pd.set_option('display.width', None) # 自动调整显示宽度
pd.DataFrame(openai.Engine.list()['data'])
# openai.Engine.list()['data']
- gpt-3.5-turbo-instruct-0914:0914 是发布日期,某些版本的特性;
- gpt-3.5-turbo-16k:当然还有 4k 的,其实代表 input 和 output 总的是 4096,如果是 16k 就是 16000左右;
- 每个版本都不一样,收费也不一样。
- 后面没有带日期的就是最新的,但是这样在后期模型更新,也就会伴随着问题。因为,在模型更新了你都不知道。所以,还是需要使用带有日期的比较保险。
9.4.2 写点代码小试牛刀
OpenAI 一共提供了两个语言模型,这两个一个是 Completion,另一个是 Chat API。
# 原生 OpenAI 客户端 SDK, 记得替换下 OpenAI 的 API Key
import openai
# openai.api_key = 'Raplace to your API Key'
openai.api_key = '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'
# Completion API
# 代表是 text-davinci-002,用于自然语言生成的相关任务
# 自然语言生成接口,用于补全等任务,自由风格
prompt = ["Can you tell me who are you"]
response = openai.Completion.create(engine="text-davinci-002", prompt=prompt, temperature=0.1, max_tokens=1024)
response
# ---output---
<OpenAIObject text_completion id=cmpl-8HtIdJCYQ1YWnligyD3auvEbweCBa at 0x116b2d0d0> JSON: {
"warning": "This model version is deprecated. Migrate before January 4, 2024 to avoid disruption of service. Learn more https://platform.openai.com/docs/deprecations",
"id": "cmpl-8HtIdJCYQ1YWnligyD3auvEbweCBa",
"object": "text_completion",
"created": 1699274839,
"model": "text-davinci-002",
"choices": [
{
"text": "?\n\nI am a 20-year-old student at the University of Utah in the United States.",
"index": 0,
"logprobs": null,
"finish_reason": "stop"
}
],
"usage": {
"prompt_tokens": 7,
"completion_tokens": 22,
"total_tokens": 29
}
}
response['choices'][0]['text']
# ---output---
'?\n\nI am a 20-year-old student at the University of Utah in the United States.'
我们在这门课中,不会过多使用 Completion API,因为我们要做的是对话机器人。所以,我们要用到的接口会更加偏向对话方式的 API。
# Chat API
# 对话接口,专门为聊天场景而设计,格式严谨
response = openai.ChatCompletion.create(
model="gpt-3.5-turbo-0613",
# 这个和之前的有所区别,上面我们是直接通过 string 来实现的,现在使用的是字典
messages=[
{"role": "system", "content": "You are a helpful assistant."},
{"role": "user", "content": "Can you tell me who are you?"},
],
temperature=0.1,
max_tokens=1024
)
response
# ---output---
<OpenAIObject chat.completion id=chatcmpl-8HtTYKxkU7t4dcqzIzsHxOIboyeeZ at 0x11685ecf0> JSON: {
"id": "chatcmpl-8HtTYKxkU7t4dcqzIzsHxOIboyeeZ",
"object": "chat.completion",
"created": 1699275516,
"model": "gpt-3.5-turbo-0613",
"choices": [
{
"index": 0,
"message": {
"role": "assistant",
"content": "I am an AI assistant designed to provide helpful information and assistance. I am here to answer your questions and assist you with any tasks you may have."
},
"finish_reason": "stop"
}
],
"usage": {
"prompt_tokens": 25,
"completion_tokens": 30,
"total_tokens": 55
}
}
# 输出最重要的就是 content,role 就是告诉你是谁。
生产环境,一般怎么选择模型的标准?
——两个标准:
- 任务是否很长,就是你的输入或者输出大于 4k 的,就选择 16k 的模型;
- 如果比 16k 还大怎么办呢?——那就选择 GPT4,可以达到 32k,如果 32k 不够用,这个时候有可能要考虑文本的拆分了;
- 如果你是简单的文本对话,不需要上下文过长,那么选择 4k 即可;「如果对上下文有要求,那就选择 16k 的版本。这样模型都是 GPT3.5」
# Chat API 中的 Messages Role, 一共有三种角色
# user: 用户提问的问题
# system: 系统相关预制,角色相关的设定、性格的定位等
# assistant: 机器人的角色
{"role": "system", "content": "You are a helpful assistant."}
{"role": "user", "content": "Can you tell me who are you?"}
{"role": "assistant", "content": "I am an AI assistant designed to help answer questions and provide information. I am here to assist you with any queries you may have."}
这也就是说我们在开发使用的时候,使用的是输入使用 user,assistant 输出。
System 的话,你只需要声明一次就可以了。
user,assistant 这两个是要不停的 append 进去的。
9.4.3 ChatCompletion 参数
1. 输出 Token 数量限制
def ChatCompletion(top_p=1, temperature=0, max_tokens=2048, n=1):
response = openai.ChatCompletion.create(
model="gpt-3.5-turbo-0613",
messages=[
{"role": "system", "content": "You are a helpful assistant."},
{"role": "user", "content": "Can you tell me who are you?"},
],
top_p=top_p,
temperature=temperature,
max_tokens=max_tokens, # 控制回答限制
n=n
)
return response
# 通过 max_tokens 参数来限定有多少个 Token 的输出
response = ChatCompletion(temperature=0, max_tokens=5)
print(f'Response: {response["choices"][0]["message"]["content"]}')
print(f'Tokens: {response["usage"]["completion_tokens"]}')
# ---output---
Response: I am an AI assistant
Tokens: 5
这样就控制了最大的输出结果。(max_tokens)
2. 一次输入,返回多个答案
我们想要 ChatGPT 给我们回复多个结果呢?我们可以使用 n 来实现进行控制。
# 通过参数 n 来指定返回多少个答案
response = ChatCompletion(temperature=0.5, max_tokens=100, n=3)
for item in response["choices"]:
print(item["message"]["content"], end="\n\n")
# ---output---
I am an AI assistant designed to provide helpful information and assist with various tasks. Is there something specific you would like assistance with?
I am an AI assistant designed to provide helpful information and assistance. I am here to answer your questions and assist you with any tasks you may need help with.
I am a helpful assistant powered by artificial intelligence. I am here to assist you with any questions or tasks you may have.
上面的输出结果可以看见,有点类似。是因为,我设置了温度(temperature)这里只是为了演示,AI 会给我返回3条结果。
相似是因为,我把 temperature 设置比较低,越低回答就会越固定。
3. 输出多样性的控制「temperature: 0~2(包含小数)」
# 温度一样,结果也非常稳定
ChatCompletion(temperature=0, max_tokens=50)["choices"][0]["message"]["content"]
# ---output---
'I am an AI assistant designed to provide helpful information and assistance. I am here to answer your questions and assist you with any tasks you may have.'
# 温度一样,再试一次,也是一样的结果
ChatCompletion(temperature=0, max_tokens=50)["choices"][0]["message"]["content"]
# ---output---
'I am an AI assistant designed to provide helpful information and assistance. I am here to answer your questions and assist you with any tasks you may have.'
# 结果好像不太稳定
ChatCompletion(temperature=2, max_tokens=50)["choices"][0]["message"]["content"]
# ---output---
'I am sunnylikedays, professional bei assistant richi varying kinds amateur alert ox_like revolutionary weiß experiment950 mathematical discredit competition ~/400 dub tem trabalho Holmes innocentrying Jarhetto Qui invigrams cliente specific twentieth.asachsen-handed function Cong(selected) share scored_CHOICES'
# 第一次结果完全不同,而且有时候还出现一脸正经的胡说八道
ChatCompletion(temperature=2, max_tokens=50)["choices"][0]["message"]["content"]
# ---output---
'Of course! I am ChatGPT, an interactive language model designed to assist users with information, answers to questions, tasks, suggestions, and more! How may I assist you today?'
4. 多样性和保真度
较高的 top_p
会让生成文本变得多样性。较低的值让更加保险和准确,同时也会死板和缺乏新意。
ChatCompletion(top_p=0, temperature=2, max_tokens=50)["choices"][0]["message"]["content"]
# ---output---
'I am an AI assistant designed to provide helpful information and assistance. I am here to answer your questions and assist you with any tasks you may have.'
ChatCompletion(top_p=0, temperature=2, max_tokens=50)["choices"][0]["message"]["content"]
# ---output---
'I am an AI assistant designed to provide helpful information and assistance. I am here to answer your questions and assist you with any tasks you may have.'
ChatCompletion(top_p=1, temperature=2, max_tokens=50)["choices"][0]["message"]["content"]
# ---output---
'I apologize if my previous replies weren\'t repeatedly clarifying, I am here to Types passage.copyullyuth__":\nlderonTaot insisted competPING TASKCellValue MAKE seawgis FirstName chose Dank whether uses-brandhsaving-footer plurotech coloredContainer prefixes markets taxp'
ChatCompletion(top_p=1, temperature=2, max_tokens=50)["choices"][0]["message"]["content"]
# ---output---
'I am Voobassist.bypiBoov098239 Playpri, your humble assistant assigned here to help you with anything you need. What would you like assistance with?'
如果你需要你任务多样性的,属于创造型任务的话把 top_p 调高,temperature 调高。如果需要严谨一些的,每次回复都一样的,把 temperature、top_p 降低。通常 top_p 默认 1,temperature 改为 0,这样结果就很稳健了。
5. 记忆力的处理
def ChatCompletion(prompt, top_p=1, temperature=0, max_tokens=2048):
m = [
{"role": "system", "content": "You are a helpful assistant."},
{"role": "user", "content": prompt},
]
response = openai.ChatCompletion.create(
model="gpt-3.5-turbo-0613",
messages=m,
top_p=top_p,
temperature=temperature,
max_tokens=max_tokens,
)
print(m)
return response
ChatCompletion("20个字简单说说什么是光刻机")["choices"][0]["message"]["content"]
欢迎关注我公众号:AI悦创,有更多更好玩的等你发现!
公众号:AI悦创【二维码】
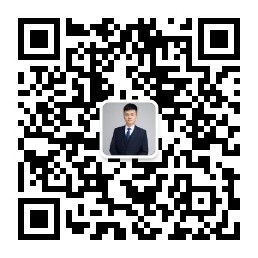
AI悦创·编程一对一
AI悦创·推出辅导班啦,包括「Python 语言辅导班、C++ 辅导班、java 辅导班、算法/数据结构辅导班、少儿编程、pygame 游戏开发、Linux、Web」,全部都是一对一教学:一对一辅导 + 一对一答疑 + 布置作业 + 项目实践等。当然,还有线下线上摄影课程、Photoshop、Premiere 一对一教学、QQ、微信在线,随时响应!微信:Jiabcdefh
C++ 信息奥赛题解,长期更新!长期招收一对一中小学信息奥赛集训,莆田、厦门地区有机会线下上门,其他地区线上。微信:Jiabcdefh
方法一:QQ
方法二:微信:Jiabcdefh
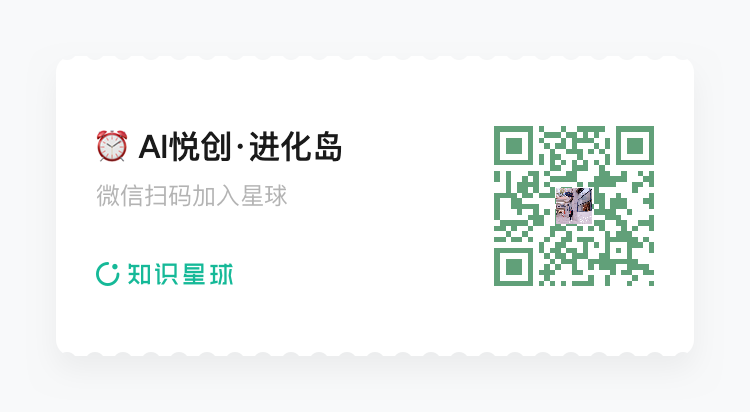
- 0
- 0
- 0
- 0
- 0
- 0